scEU-seq organoid
This tutorial uses the intestine organoid data from Battich, et al (2020). This tutorial is the second one of the two tutorials for demonstrating how dynamo can use used to analyze the scEU-seq data. Please refer the cell cycle tutorial for details on how to analyze the cell cycle dataset.
# !pip install git+https://github.com/aristoteleo/dynamo-release@master
import warnings
warnings.filterwarnings('ignore')
import dynamo as dyn
import anndata
import pandas as pd
import numpy as np
import scipy.sparse
from anndata import AnnData
from scipy.sparse import csr_matrix
# dyn.get_all_dependencies_version()
Load data
organoid = dyn.sample_data.scEU_seq_organoid()
|-----> Downloading scEU_seq data
|-----> Downloading data to ./data/organoid.h5ad
|-----> in progress: 99.0108%|-----> [download] completed [17.9992s]
organoid
AnnData object with n_obs × n_vars = 3831 × 9157
obs: 'well_id', 'batch_id', 'treatment_id', 'log10_gfp', 'rotated_umap1', 'rotated_umap2', 'som_cluster_id', 'monocle_branch_id', 'monocle_pseudotime', 'exp_type', 'time'
var: 'ID', 'NAME'
layers: 'sl', 'su', 'ul', 'uu'
# mapping:
cell_mapper = {
'1': 'Enterocytes',
'2': 'Enterocytes',
'3': 'Enteroendocrine',
'4': 'Enteroendocrine progenitor',
'5': 'Tuft cells',
'6': 'TA cells',
'7': 'TA cells',
'8': 'Stem cells',
'9': 'Paneth cells',
'10': 'Goblet cells',
'11': 'Stem cells',
}
organoid.obs['cell_type'] = organoid.obs.som_cluster_id.map(cell_mapper).astype('str')
typical dynamo analysis workflow
dyn.pl.basic_stats(organoid)
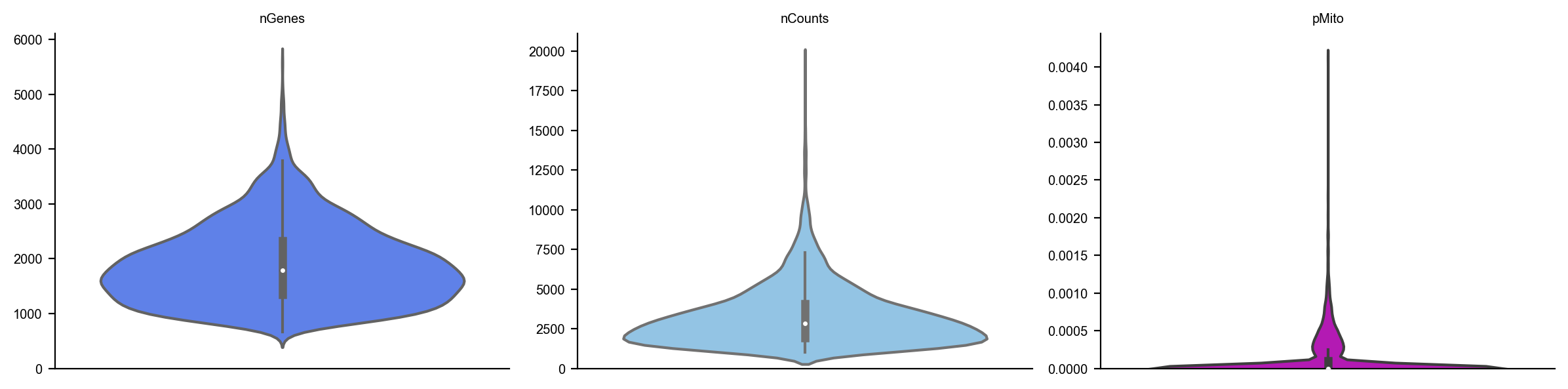
organoid
AnnData object with n_obs × n_vars = 3831 × 9157
obs: 'well_id', 'batch_id', 'treatment_id', 'log10_gfp', 'rotated_umap1', 'rotated_umap2', 'som_cluster_id', 'monocle_branch_id', 'monocle_pseudotime', 'exp_type', 'time', 'cell_type', 'nGenes', 'nCounts', 'pMito'
var: 'ID', 'NAME', 'nCells', 'nCounts'
layers: 'sl', 'su', 'ul', 'uu'
organoid.obs
well_id | batch_id | treatment_id | log10_gfp | rotated_umap1 | rotated_umap2 | som_cluster_id | monocle_branch_id | monocle_pseudotime | exp_type | time | cell_type | nGenes | nCounts | pMito | |
---|---|---|---|---|---|---|---|---|---|---|---|---|---|---|---|
1 | 14 | 01 | Pulse_120 | 12.8929281522 | 23.0662174225 | -3.47039175034 | 6 | 2 | 6.08688834859 | Pulse | 120 | TA cells | 1054 | 1426.0 | 0.0 |
2 | 15 | 01 | Pulse_120 | 5.85486775252 | 25.710735321 | -1.31835341454 | 2 | 2 | 9.14740876358 | Pulse | 120 | Enterocytes | 1900 | 3712.0 | 0.0 |
3 | 16 | 01 | Pulse_120 | 7.45690471634 | 26.7709560394 | -1.06682777405 | 2 | 2 | 9.69134627386 | Pulse | 120 | Enterocytes | 2547 | 6969.0 | 0.0 |
4 | 17 | 01 | Pulse_120 | 94.2814535609 | 21.2927913666 | 0.0159659013152 | 11 | 2 | 4.2635104705 | Pulse | 120 | Stem cells | 1004 | 1263.0 | 0.0 |
5 | 21 | 01 | Pulse_120 | 47.1412266395 | 19.9096126556 | 0.884054124355 | 11 | 1 | 2.62248093423 | Pulse | 120 | Stem cells | 927 | 1144.0 | 0.0 |
... | ... | ... | ... | ... | ... | ... | ... | ... | ... | ... | ... | ... | ... | ... | ... |
3827 | 378 | 12 | Pulse_120 | 32.496816667 | 20.7663478851 | -3.72811675072 | 8 | 3 | 7.32939908351 | Pulse | 120 | Stem cells | 2268 | 3918.0 | 0.0 |
3828 | 379 | 12 | Pulse_120 | 78.1198193763 | 20.1073760986 | -2.65023303032 | 8 | 3 | 5.10436147713 | Pulse | 120 | Stem cells | 2131 | 3619.0 | 0.0 |
3829 | 380 | 12 | Pulse_120 | 53.249846399 | 20.1618804932 | -3.83158016205 | 8 | 3 | 6.43742448317 | Pulse | 120 | Stem cells | 2141 | 3603.0 | 0.0 |
3830 | 381 | 12 | Pulse_dmso | 16.7070737849 | 15.4272613525 | -2.15779066086 | 10 | 1 | 0.657880511889 | Pulse | dmso | Goblet cells | 1158 | 1683.0 | 0.0 |
3831 | 383 | 12 | Pulse_dmso | 93.3716092195 | 21.5953540802 | -3.90664196014 | 6 | 2 | 4.81727202212 | Pulse | dmso | TA cells | 1374 | 1838.0 | 0.0 |
3831 rows × 15 columns
organoid.obs.groupby(['exp_type', 'time']).agg('count')
well_id | batch_id | treatment_id | log10_gfp | rotated_umap1 | rotated_umap2 | som_cluster_id | monocle_branch_id | monocle_pseudotime | cell_type | nGenes | nCounts | pMito | ||
---|---|---|---|---|---|---|---|---|---|---|---|---|---|---|
exp_type | time | |||||||||||||
Chase | 0 | 660 | 660 | 660 | 660 | 660 | 660 | 660 | 660 | 660 | 660 | 660 | 660 | 660 |
45 | 821 | 821 | 821 | 821 | 821 | 821 | 821 | 821 | 821 | 821 | 821 | 821 | 821 | |
120 | 0 | 0 | 0 | 0 | 0 | 0 | 0 | 0 | 0 | 0 | 0 | 0 | 0 | |
360 | 646 | 646 | 646 | 646 | 646 | 646 | 646 | 646 | 646 | 646 | 646 | 646 | 646 | |
dmso | 0 | 0 | 0 | 0 | 0 | 0 | 0 | 0 | 0 | 0 | 0 | 0 | 0 | |
Pulse | 0 | 0 | 0 | 0 | 0 | 0 | 0 | 0 | 0 | 0 | 0 | 0 | 0 | 0 |
45 | 0 | 0 | 0 | 0 | 0 | 0 | 0 | 0 | 0 | 0 | 0 | 0 | 0 | |
120 | 1373 | 1373 | 1373 | 1373 | 1373 | 1373 | 1373 | 1373 | 1373 | 1373 | 1373 | 1373 | 1373 | |
360 | 0 | 0 | 0 | 0 | 0 | 0 | 0 | 0 | 0 | 0 | 0 | 0 | 0 | |
dmso | 331 | 331 | 331 | 331 | 331 | 331 | 331 | 331 | 331 | 331 | 331 | 331 | 331 |
adata = organoid.copy()
adata.obs.time = adata.obs.time.astype('str')
adata.obs.loc[adata.obs['time'] == 'dmso', 'time'] = -1
adata.obs['time'] = adata.obs['time'].astype(float)
adata = adata[adata.obs.time != -1, :]
adata = adata[adata.obs.exp_type == 'Pulse', :]
adata.layers['new'], adata.layers['total'] = adata.layers['ul'] + adata.layers['sl'], adata.layers['su'] + adata.layers['sl'] + adata.layers['uu'] + adata.layers['ul']
del adata.layers['uu'], adata.layers['ul'], adata.layers['su'], adata.layers['sl']
# dyn.pp.recipe_monocle(adata, n_top_genes=1000, total_layers=False)
preprocessor = dyn.pp.Preprocessor(cell_cycle_score_enable=True)
preprocessor.config_monocle_recipe(adata, n_top_genes=1000)
preprocessor.filter_cells_by_outliers_kwargs["keep_filtered"] = True
preprocessor.preprocess_adata_monocle(adata)
dyn.pl.basic_stats(adata)
dyn.pl.show_fraction(organoid)
|-----> Running monocle preprocessing pipeline...
|-----? No 'tkey' value was given despite 'tkey' information in the adata, so we will use 'time' in the adata as the default.
|-----------> filtered out 0 outlier cells
|-----------> filtered out 815 outlier genes
|-----> PCA dimension reduction
|-----> <insert> X_pca to obsm in AnnData Object.
|-----> computing cell phase...
|-----> [Cell Phase Estimation] completed [20.6928s]
|-----> [Cell Cycle Scores Estimation] completed [0.0563s]
|-----> [Preprocessor-monocle] completed [1.9278s]
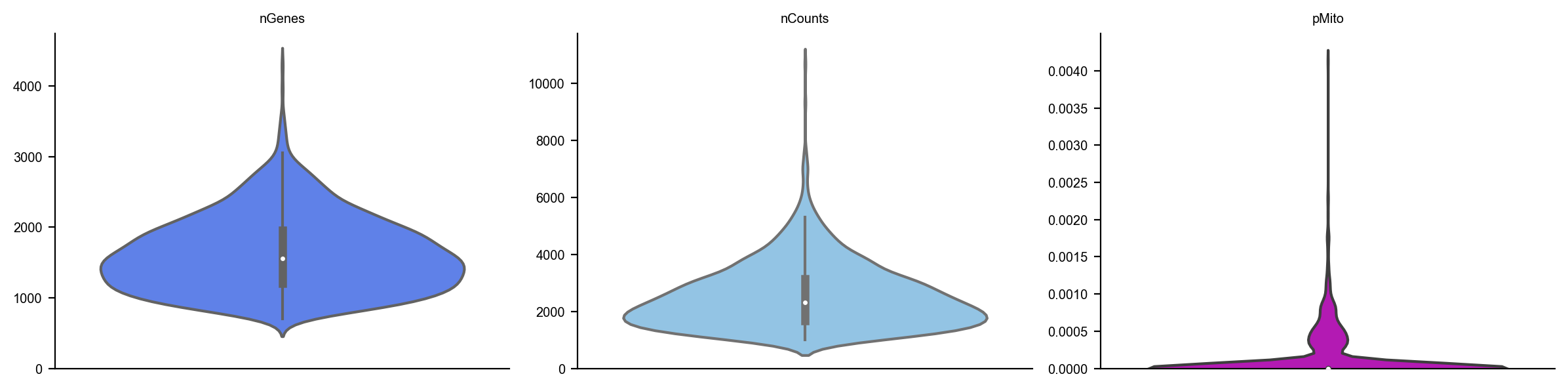

adata.obs.time = adata.obs.time/60
adata.obs.time = adata.obs.time.astype('float')
dyn.tl.dynamics(adata, model='deterministic', tkey='time', assumption_mRNA='ss')
dyn.tl.reduceDimension(adata)
|-----> dynamics_del_2nd_moments_key is None. Using default value from DynamoAdataConfig: dynamics_del_2nd_moments_key=False |-----------> removing existing M layers:[]... |-----------> making adata smooth... |-----> calculating first/second moments... |-----> [moments calculation] completed [6.7972s] |-----? Your adata only has labeling data, but NTR_vel is set to be False. Dynamo will reset it to True to enable this analysis.
estimating gamma: 100%|█████████████████████| 1000/1000 [00:10<00:00, 91.65it/s]
|-----> retrieve data for non-linear dimension reduction...
|-----> [UMAP] using X_pca with n_pca_components = 30
|-----> <insert> X_umap to obsm in AnnData Object.
|-----> [UMAP] completed [7.7419s]
dyn.tl.cell_velocities(adata, ekey='M_t', vkey='velocity_T', enforce=True)
|-----> incomplete neighbor graph info detected: connectivities and distances do not exist in adata.obsp, indices not in adata.uns.neighbors.
|-----> Neighbor graph is broken, recomputing....
|-----> Start computing neighbor graph...
|-----------> X_data is None, fetching or recomputing...
|-----> fetching X data from layer:None, basis:pca
|-----> method arg is None, choosing methods automatically...
|-----------> method ball_tree selected
|-----> [calculating transition matrix via pearson kernel with sqrt transform.] in progress: 100.0000%|-----> [calculating transition matrix via pearson kernel with sqrt transform.] completed [2.5574s]
|-----> [projecting velocity vector to low dimensional embedding] in progress: 100.0000%|-----> [projecting velocity vector to low dimensional embedding] completed [0.2453s]
AnnData object with n_obs × n_vars = 1373 × 9157
obs: 'well_id', 'batch_id', 'treatment_id', 'log10_gfp', 'rotated_umap1', 'rotated_umap2', 'som_cluster_id', 'monocle_branch_id', 'monocle_pseudotime', 'exp_type', 'time', 'cell_type', 'nGenes', 'nCounts', 'pMito', 'pass_basic_filter', 'total_Size_Factor', 'initial_total_cell_size', 'new_Size_Factor', 'initial_new_cell_size', 'Size_Factor', 'initial_cell_size', 'ntr', 'cell_cycle_phase'
var: 'ID', 'NAME', 'nCells', 'nCounts', 'pass_basic_filter', 'log_m', 'log_cv', 'score', 'frac', 'use_for_pca', 'ntr', 'alpha', 'beta', 'gamma', 'half_life', 'alpha_b', 'alpha_r2', 'gamma_b', 'gamma_r2', 'gamma_logLL', 'delta_b', 'delta_r2', 'bs', 'bf', 'uu0', 'ul0', 'su0', 'sl0', 'U0', 'S0', 'total0', 'beta_k', 'gamma_k', 'use_for_dynamics', 'use_for_transition'
uns: 'pp', 'velocyto_SVR', 'feature_selection', 'PCs', 'explained_variance_ratio_', 'pca_mean', 'cell_phase_genes', 'dynamics', 'neighbors', 'umap_fit', 'grid_velocity_umap'
obsm: 'X_pca', 'cell_cycle_scores', 'X_umap', 'velocity_umap'
varm: 'alpha'
layers: 'new', 'total', 'X_total', 'X_new', 'M_t', 'M_tt', 'M_n', 'M_tn', 'M_nn', 'velocity_N', 'velocity_T'
obsp: 'moments_con', 'distances', 'connectivities', 'pearson_transition_matrix'
adata.obsm['X_umap_ori'] = adata.obs.loc[:, ['rotated_umap1', 'rotated_umap2']].values.astype(float)
Visualize time-resolved vector flow learned with dynamo
dyn.tl.cell_velocities(adata, basis='umap_ori')
dyn.pl.streamline_plot(adata, color='cell_type', basis='umap_ori')
Using existing pearson_transition_matrix found in .obsp.
|-----> [projecting velocity vector to low dimensional embedding] in progress: 100.0000%|-----> [projecting velocity vector to low dimensional embedding] completed [0.2327s]
|-----------> plotting with basis key=X_umap_ori
|-----------> skip filtering cell_type by stack threshold when stacking color because it is not a numeric type
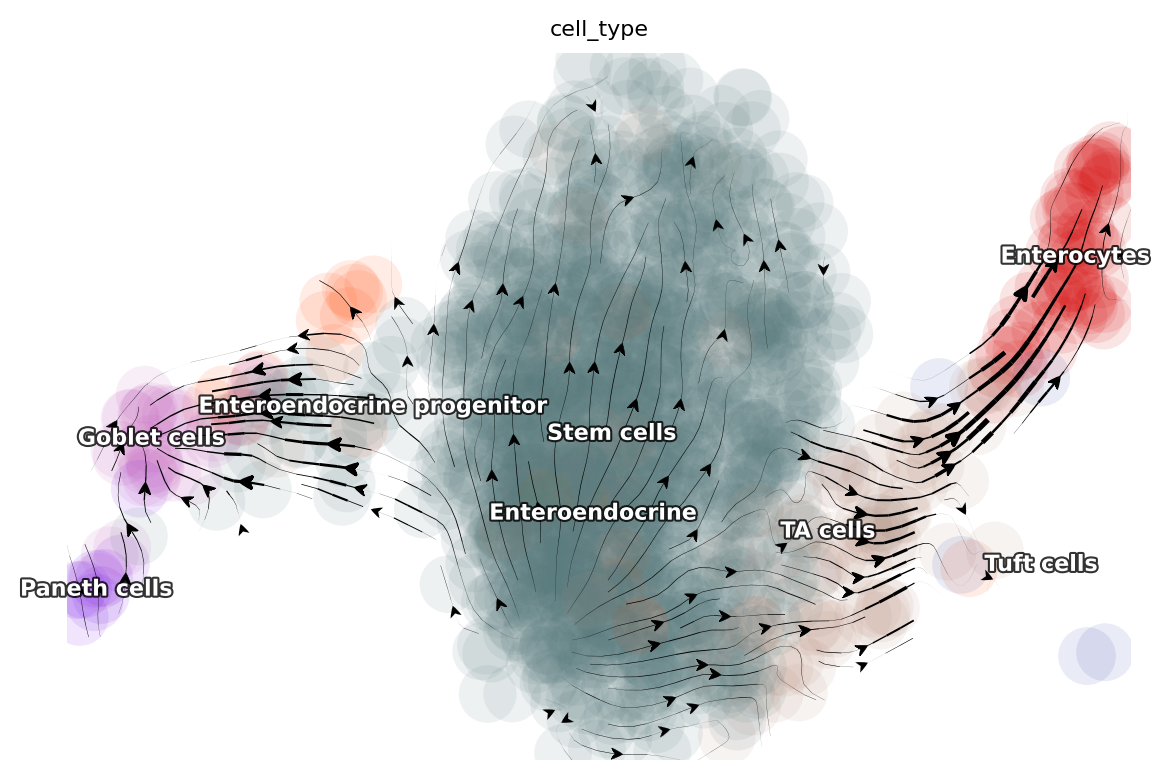
dyn.pl.streamline_plot(adata, color='cell_cycle_phase', basis='umap_ori')
|-----------> plotting with basis key=X_umap_ori
|-----------> skip filtering cell_cycle_phase by stack threshold when stacking color because it is not a numeric type
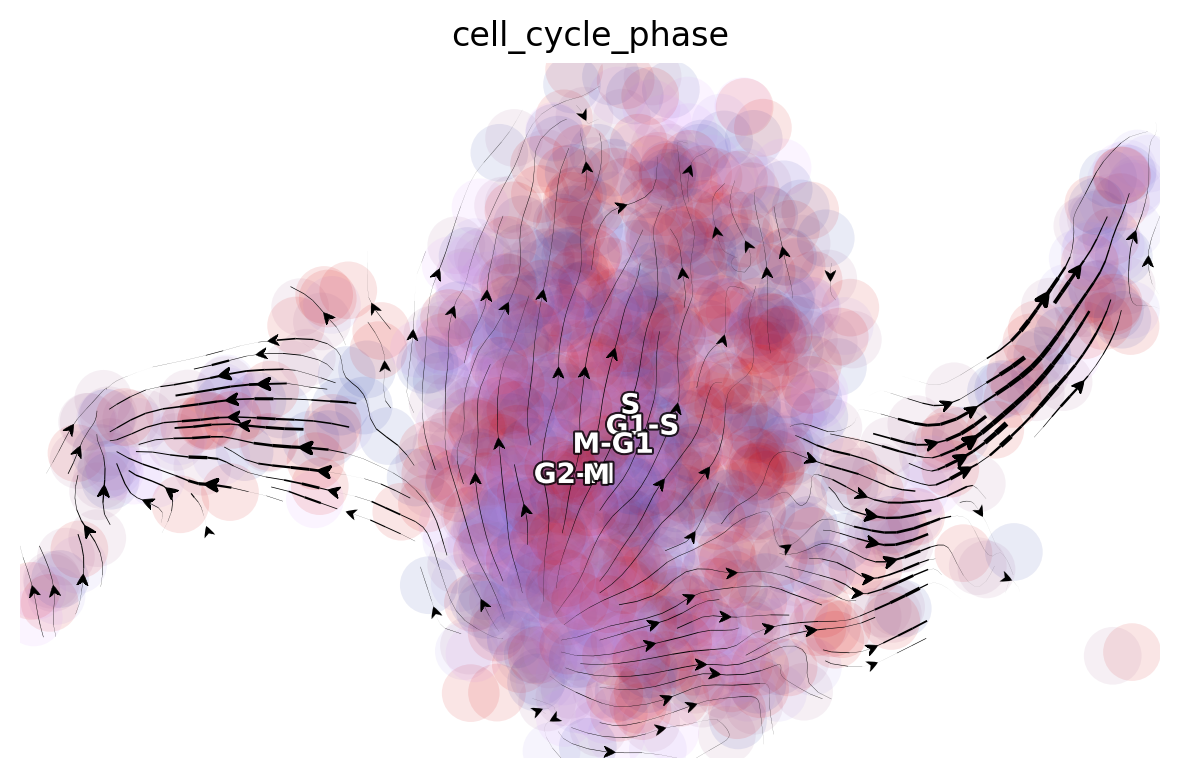
adata.var_names[adata.var.use_for_transition][:5]
Index(['Cdc45', 'Brat1', 'Ccnd2', 'Ckmt1', 'Pdgfb'], dtype='object')
dyn.pl.phase_portraits(adata, genes=['Brat1', 'Ccnd2', 'Ckmt1', 'Pdgfb', 'Gpa33'],
color='som_cluster_id', basis='umap_ori')
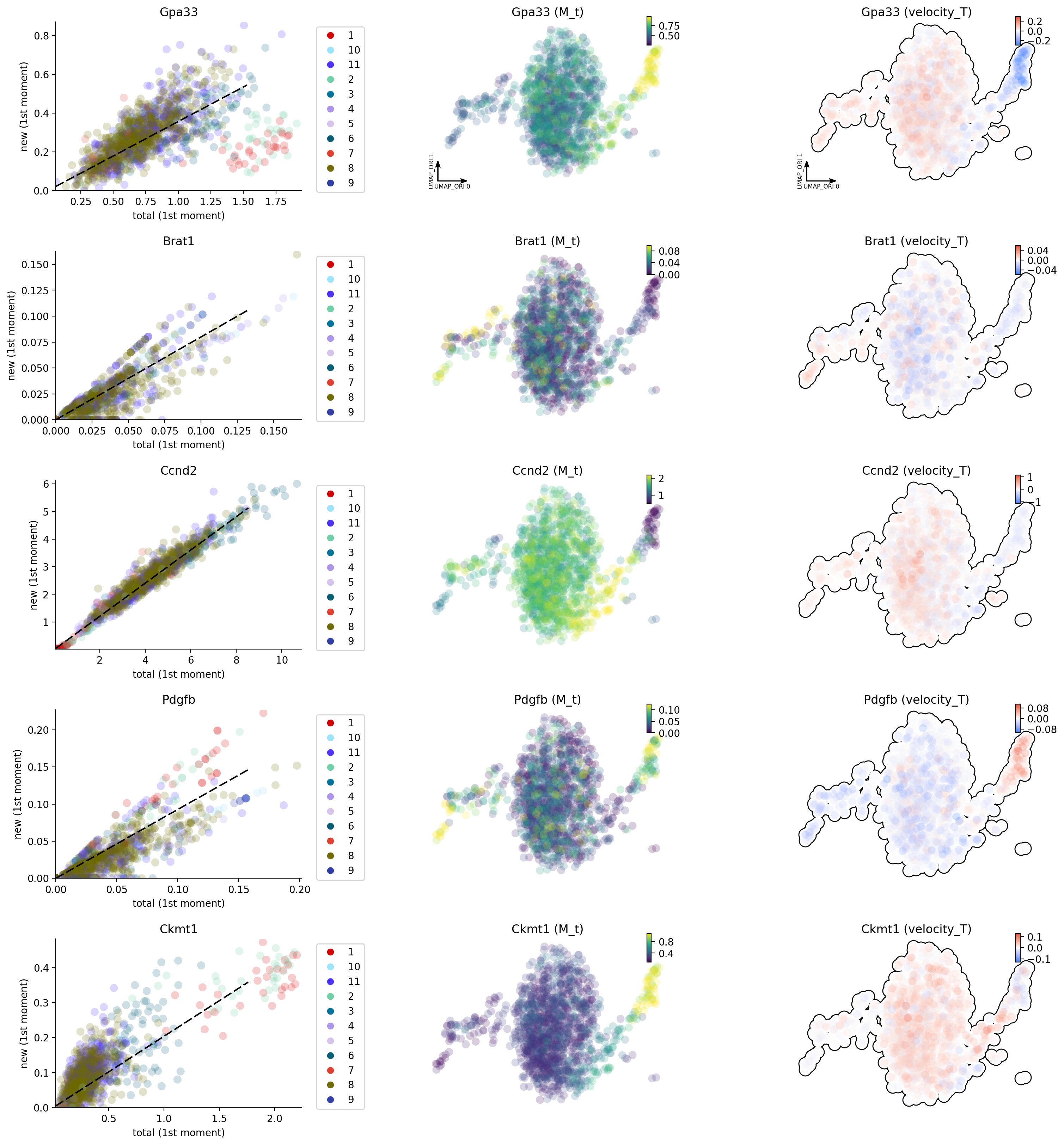
Animate intestine organoid differentiation
dyn.vf.VectorField(adata, basis='umap_ori')
|-----> VectorField reconstruction begins...
|-----> Retrieve X and V based on basis: UMAP_ORI.
Vector field will be learned in the UMAP_ORI space.
|-----> Generating high dimensional grids and convert into a row matrix.
|-----> Learning vector field with method: sparsevfc.
|-----> [SparseVFC] begins...
|-----> Sampling control points based on data velocity magnitude...
|-----> [SparseVFC] completed [0.1492s]
|-----> [VectorField] completed [0.1768s]
progenitor = adata.obs_names[adata.obs.cell_type == 'Stem cells']
len(progenitor)
1146
np.random.seed(19491001)
from matplotlib import animation
info_genes = adata.var_names[adata.var.use_for_transition]
dyn.pd.fate(adata, basis='umap_ori', init_cells=progenitor[:100], interpolation_num=100, direction='forward',
inverse_transform=False, average=False)
integration with ivp solver: 100%|████████████| 100/100 [00:08<00:00, 11.67it/s]
uniformly sampling points along a trajectory: 100%|█| 100/100 [00:00<00:00, 550.
AnnData object with n_obs × n_vars = 1373 × 9157
obs: 'well_id', 'batch_id', 'treatment_id', 'log10_gfp', 'rotated_umap1', 'rotated_umap2', 'som_cluster_id', 'monocle_branch_id', 'monocle_pseudotime', 'exp_type', 'time', 'cell_type', 'nGenes', 'nCounts', 'pMito', 'pass_basic_filter', 'total_Size_Factor', 'initial_total_cell_size', 'new_Size_Factor', 'initial_new_cell_size', 'Size_Factor', 'initial_cell_size', 'ntr', 'cell_cycle_phase', 'control_point_umap_ori', 'inlier_prob_umap_ori', 'obs_vf_angle_umap_ori'
var: 'ID', 'NAME', 'nCells', 'nCounts', 'pass_basic_filter', 'log_m', 'log_cv', 'score', 'frac', 'use_for_pca', 'ntr', 'alpha', 'beta', 'gamma', 'half_life', 'alpha_b', 'alpha_r2', 'gamma_b', 'gamma_r2', 'gamma_logLL', 'delta_b', 'delta_r2', 'bs', 'bf', 'uu0', 'ul0', 'su0', 'sl0', 'U0', 'S0', 'total0', 'beta_k', 'gamma_k', 'use_for_dynamics', 'use_for_transition'
uns: 'pp', 'velocyto_SVR', 'feature_selection', 'PCs', 'explained_variance_ratio_', 'pca_mean', 'cell_phase_genes', 'dynamics', 'neighbors', 'umap_fit', 'grid_velocity_umap', 'grid_velocity_umap_ori', 'cell_type_colors', 'cell_cycle_phase_colors', 'VecFld_umap_ori', 'fate_umap_ori'
obsm: 'X_pca', 'cell_cycle_scores', 'X_umap', 'velocity_umap', 'X_umap_ori', 'velocity_umap_ori', 'velocity_umap_ori_SparseVFC', 'X_umap_ori_SparseVFC'
varm: 'alpha'
layers: 'new', 'total', 'X_total', 'X_new', 'M_t', 'M_tt', 'M_n', 'M_tn', 'M_nn', 'velocity_N', 'velocity_T'
obsp: 'moments_con', 'distances', 'connectivities', 'pearson_transition_matrix'
%%capture
import matplotlib.pyplot as plt
fig, ax = plt.subplots()
ax = dyn.pl.topography(adata, basis='umap_ori', color='cell_type', ax=ax, save_show_or_return='return', figsize=(24, 24))
ax.set_aspect(0.8)
|-----> VectorField reconstruction begins...
|-----> Retrieve X and V based on basis: UMAP.
Vector field will be learned in the UMAP space.
|-----> Generating high dimensional grids and convert into a row matrix.
|-----> Learning vector field with method: sparsevfc.
|-----> [SparseVFC] begins...
|-----> Sampling control points based on data velocity magnitude...
|-----> [SparseVFC] completed [0.1355s]
|-----> Mapping topography...
|-----> [VectorField] completed [1.8211s]
|-----------> plotting with basis key=X_umap_ori
|-----------> skip filtering cell_type by stack threshold when stacking color because it is not a numeric type
%%capture
adata.obs['time'] = adata.obs.time.astype('float')
instance = dyn.mv.StreamFuncAnim(adata=adata, basis='umap_ori', color='cell_type', ax=ax)
|-----? the number of cell states with fate prediction is more than 50. You may want to lower the max number of cell states to draw via cell_states argument.
|-----------> plotting with basis key=X_umap_ori
|-----------> skip filtering cell_type by stack threshold when stacking color because it is not a numeric type